Understanding Data Annotation in Machine Learning
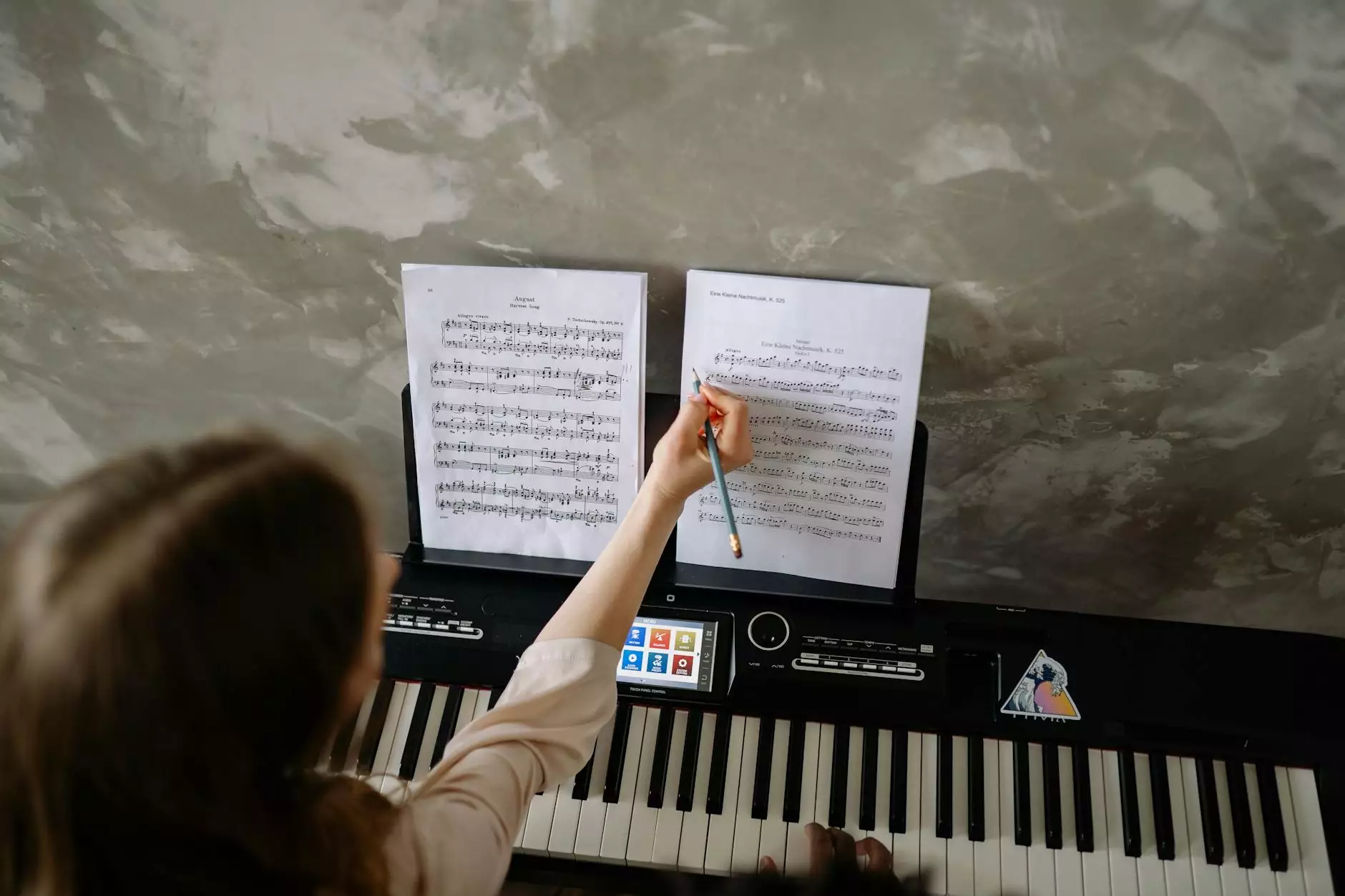
In today's rapidly evolving technological landscape, data annotation has emerged as a cornerstone of machine learning (ML). This vital process involves labeling data to provide context for algorithms, thus enhancing the accuracy of machine learning models. As businesses increasingly rely on automated solutions to improve efficiencies, understanding the intricacies of data annotation becomes essential.
The Significance of Data Annotation
Without precise data annotation, machine learning models cannot learn effectively from raw data. This process is instrumental for various applications, from computer vision and natural language processing to automated reasoning systems. The quality of the annotated data directly affects the model's performance, underscoring the importance of robust annotation practices.
What is Data Annotation?
Data annotation is the method of labeling datasets to train algorithms for machine learning. This activity can take numerous forms, such as:
- Image Annotation: Labeling objects in images for computer vision tasks.
- Text Annotation: Identifying entities in text documents for sentiment analysis.
- Audio Annotation: Tagging sounds or speech for tasks in voice recognition systems.
The Role of Data Annotation in Machine Learning Development
Properly annotated data allows machine learning models to learn patterns and make predictions. The ML lifecycle is heavily reliant on both the quality and the quantity of labeled data. Here are some critical aspects of this role:
1. Improving Accuracy
The difference between a mediocre and an outstanding machine learning model often lies in the quality of data annotation. Well-annotated datasets enable algorithms to recognize features and relationships correctly, leading to more accurate predictions.
2. Saving Time and Resources
Investing in professional data annotation can significantly reduce the time and resources required for model training. Efficient annotation services help organizations avoid common pitfalls that delay project timelines, such as re-annotations due to poor labeling.
3. Enhancing Performance
Data annotation fuels the performance of machine learning applications. Systems with accurately labeled datasets outperform those built with unstructured data. Enhanced performance translates to better user experiences and ultimately leads to higher customer satisfaction.
Challenges in Data Annotation
Although data annotation is crucial, it comes with its own set of challenges:
- Subjectivity: Different annotators may interpret data differently, leading to inconsistencies.
- Scalability: As datasets grow, ensuring quality across massive volumes of data becomes tougher.
- Cost: High-quality annotation requires significant investment, which may deter some businesses.
Keymakr: Leading the Way in Data Annotation Services
For businesses focusing on Home Services and Keys & Locksmiths, integrating data annotation services can streamline operations and enhance service delivery. Keymakr.com stands out as a pioneer in providing data annotation solutions, specifically tailored to meet industry needs.
Why Choose Keymakr?
Keymakr offers a unique blend of technology and expertise designed to facilitate effective data annotation. Here’s why Keymakr is a preferred choice:
- Expertise: A team of seasoned professionals ensures that the data annotation process meets the highest standards of accuracy.
- Custom Solutions: Keymakr understands the specific needs of the locksmith and home services industry, providing tailored solutions.
- Efficiency: With advanced tools and techniques, Keymakr minimizes turnaround times while maintaining quality.
- Affordability: Competitive pricing for comprehensive data annotation services without compromising quality.
Integrating Data Annotation into Your Business Model
For businesses in the locksmith and home services sectors, leveraging data annotation effectively can yield transformative results. Here are steps to integrate this process into your operations:
1. Identify Your Needs
Assess your business's requirements and pinpoint the types of data you wish to annotate. This could range from images of locks to customer service interactions.
2. Choose the Right Service Provider
Selecting a reliable data annotation provider, such as Keymakr, is fundamental. Look for providers that specialize in your industry's specific needs.
3. Implement Quality Controls
Establish quality control measures to ensure that data annotation remains consistent and accurate. Regular audits and feedback loops can help maintain high standards.
4. Monitor and Optimize
Continuously monitor the impact of annotated data on your business outcomes. Utilize analytics to make informed adjustments and improvements over time.
The Future of Data Annotation in Machine Learning
As machine learning continues to evolve, the role of data annotation will likely become even more critical. Advances in ML algorithms will necessitate larger and more complex datasets, further emphasizing the importance of precise annotation techniques.
Trends to Watch
Here are some trends to consider as we look ahead:
- Automation: The rise of AI-assisted annotation tools that streamline the process.
- Real-time Annotation: Methods that allow for on-the-fly data annotation during the data collection process.
- Increased Demand: Growing reliance on ML technologies in various sectors will drive demand for high-quality annotated data.
Conclusion
In conclusion, data annotation is a vital component of machine learning that cannot be overlooked. For businesses, especially those in the home services and locksmith industries like Keymakr, integrating effective data annotation practices can lead to significant advantages, including improved efficiency, reduced costs, and enhanced customer satisfaction. By leveraging professional data annotation services, companies can ensure they remain competitive in an increasingly automated world.
The future is bright for businesses that embrace data annotation, providing a robust foundation for sophisticated machine learning solutions. The earlier organizations acknowledge the value of proper data labeling, the greater their potential for success in harnessing the power of machine learning.
data annotation machine learning